AI-Driven RAN: Transforming Network Operations for the Future
Challenges Facing Mobile Network Operators (MNOs)
Challenges Facing Mobile Network Operators (MNOs)
As mobile networks evolve to support increasing data demand, Mobile Network Operators (MNOs) face several critical challenges:
1. Rising CAPEX Due to Network Expansions
With the rollout of 5G and upcoming 6G advancements, MNOs must invest heavily in network expansion, including:
- Deploying new sites to enhance coverage and capacity.
- Upgrading existing infrastructure to support new technologies.
- Investing in advanced hardware, software, and spectrum licenses.
2. Growing Network Complexity
As networks integrate multiple generations of technology (2G, 3G, 4G, 5G, and soon 6G), managing this complexity becomes a major challenge. Key concerns include:
- Optimizing the placement of new sites to maximize coverage and efficiency.
- Choosing the right hardware, licenses, and features to balance performance and cost.
- Ensuring seamless interworking between legacy and new network elements.
3. Increasing OPEX Due to Operations and Maintenance
Operational expenditures continue to rise due to:
- The increasing number of managed services personnel and field engineers.
- The complexity of maintaining multi-layer, multi-vendor networks.
- The need for continuous network optimization to ensure service quality.
- Rising Energy Costs: Powering an expanding network infrastructure requires substantial energy consumption, and increasing energy prices put further pressure on operational budgets. AI-driven solutions can optimize power usage, reduce waste, and shift energy consumption to off-peak times where feasible.
4. Competitive Pressures in Customer Experience & Network Quality
MNOs are not only competing on price and service offerings but also on:
- Network Quality: Coverage, speed, and reliability.
- Customer Experience: Personalized and high-quality connectivity.
- Operational Efficiency: Cost-effective operations that enhance profitability.
The Concept of AI in RAN
To address these challenges, AI-driven Radio Access Networks (AI-RAN) emerge as a key enabler. AI-RAN leverages artificial intelligence and machine learning to:
- Optimize network planning and resource allocation.
- Automate operations, reducing manual interventions.
- Enhance predictive maintenance to prevent failures before they occur.
- Improve energy efficiency by dynamically adjusting power consumption based on traffic demand.
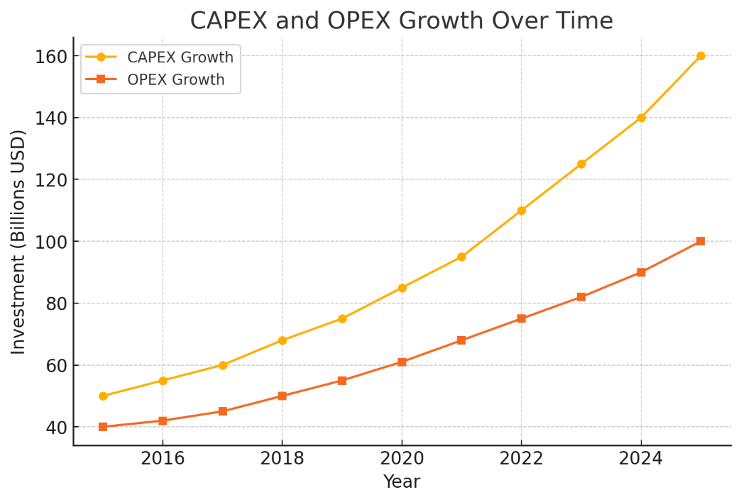
Different AI-RAN Methodologies
- AI and RAN
- AI and RAN (also referred to as AI with RAN): using a common shared infrastructure to run both AI and workloads, with the goal to maximize utilization, lower Total Cost of Ownership (TCO) and generate new AI-driven revenue opportunities.
- AI is used as an external tool for decision-making and analytics without direct integration into the RAN architecture.
- Example: AI-driven network planning tools that assist in site selection and spectrum allocation.
- AI on RAN
- AI on RAN: enabling AI services on RAN at the network edge to increase operational efficiency and offer new services to mobile users. This turns the RAN from a cost centre to a revenue source.
- AI is embedded within the RAN system to enhance real-time decision-making.
- Example: AI-powered self-optimizing networks (SON) that adjust parameters dynamically to improve network performance.
- AI for RAN
- AI for RAN: advancing RAN capabilities through embedding AI/ML models, algorithms and neural networks into the radio signal processing layer to improve spectral efficiency, radio coverage, capacity and performance.
- AI is leveraged to redesign RAN architecture for autonomous and intelligent network operations.
- Example: AI-native Open RAN solutions that enable dynamic reconfiguration of network functions.
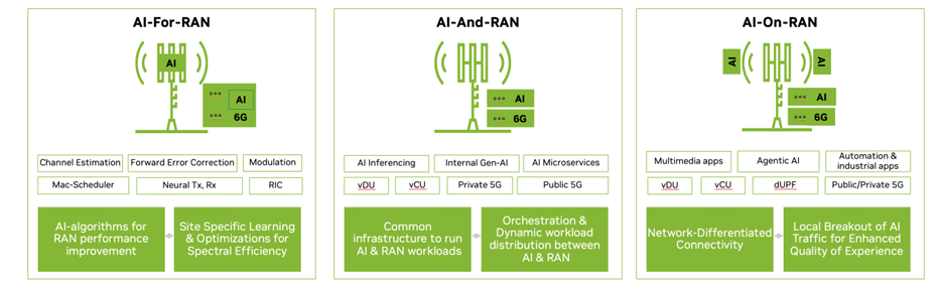
Source is NVidia AI-RAN: Artificial Intelligence – Radio Access Networks Document.
Organizations and Standardization Bodies Focusing on AI-RAN
Several industry bodies and alliances are driving AI adoption in RAN, including:
- O-RAN Alliance: Developing AI-native Open RAN architectures.
- 3GPP: Standardizing AI/ML applications in RAN.
- ETSI (European Telecommunications Standards Institute): Working on AI-powered network automation.
- ITU (International Telecommunication Union): AI for good to promote the AI use cases
- GSMA: Promoting AI-driven innovations for future networks.
- Global Telco AI Alliance: A collaboration among leading telecom operators to advance AI integration in network operations and RAN management.
AI-RAN Use Cases
- Intelligent Network Planning
- AI-driven tools analyse coverage gaps and predict optimal site locations for new deployments.
- Uses geospatial and traffic data to optimize CAPEX investments.
- Improves network rollout efficiency by identifying areas with the highest potential return on investment.
- Automated Network Optimization
- AI-powered SON dynamically adjusts network parameters.
- Enhances performance by minimizing congestion and interference.
- Predicts and mitigates traffic spikes in real-time, improving service stability.
- Predictive Maintenance
- AI detects anomalies in hardware and predicts failures before they happen.
- Uses machine learning models to analyze historical data and identify patterns leading to failures.
- Reduces downtime and minimizes maintenance costs by enabling proactive issue resolution.
- Energy Efficiency Optimization
- AI adjusts power consumption based on real-time traffic patterns.
- Identifies opportunities for network elements to enter low-power modes during off-peak hours.
- Leads to significant OPEX savings and a reduced carbon footprint by optimizing renewable energy integration.
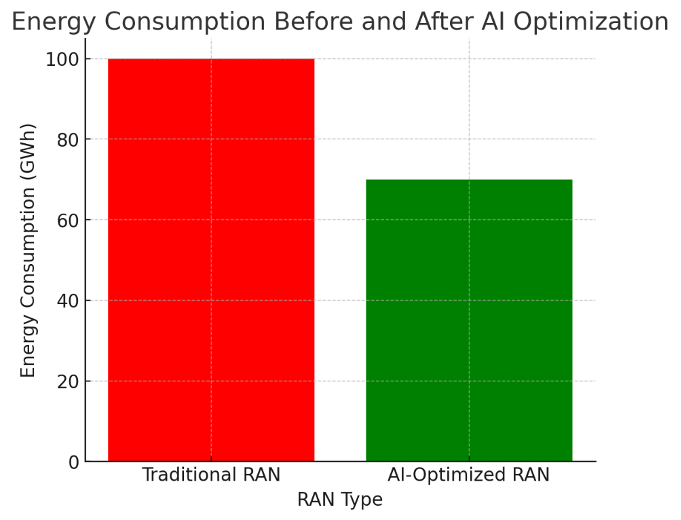
- Enhanced Customer Experience Management
- AI-driven analytics personalize network performance based on user behavior.
- Predicts and prioritizes network resources for latency-sensitive applications like gaming and video streaming.
- Uses AI-driven call quality analysis to detect and rectify issues before customers notice degradation.
- AI-Driven Interference Management
- AI models analyze interference patterns and dynamically adjust power levels and beamforming strategies.
- Reduces interference between cells and enhances spectral efficiency, especially in dense urban areas.
- Supply Chain and Inventory Optimization
- AI helps predict hardware and component needs based on network demand forecasts.
- Reduces overstocking and minimizes delays by ensuring the right components are available when needed.
- AI-Driven Beamforming Management
- AI optimizes beamforming parameters to improve signal strength and reduce interference.
- Dynamically adjusts beam directions based on real-time user movement and network conditions.
- Enhances network coverage and capacity, particularly in urban and high-density environments.
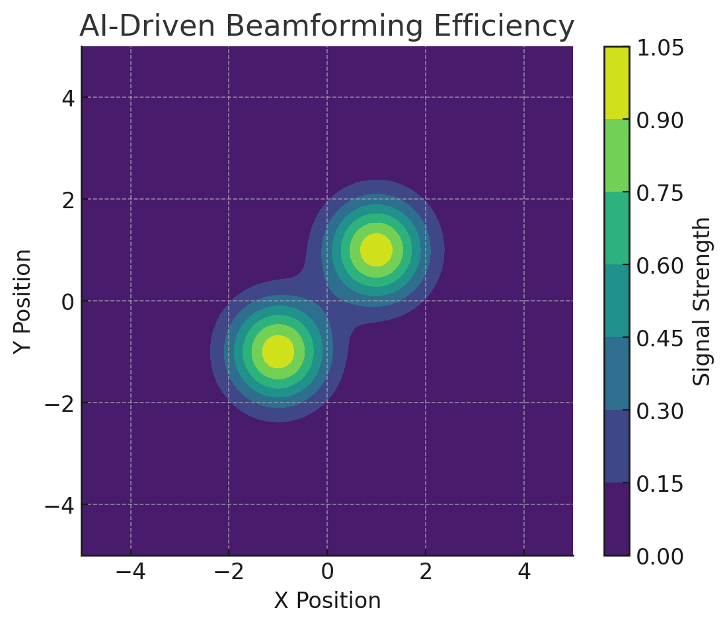
Conclusion
AI is revolutionizing RAN by enhancing efficiency, reducing costs, and improving network performance. As AI adoption in RAN continues to grow, MNOs can expect increased automation, better customer experiences, and more cost-effective network operations. The journey toward AI-driven RAN is not just an evolution—it is a necessity for the future of mobile networks.
To further illustrate these advancements, incorporating graphs that highlight AI’s impact on OPEX reduction, predictive maintenance efficiency, and energy savings will help visualize the benefits AI brings to RAN operations.
Prepared By: Abdelrahman Fady | CTO | Digis Squared