AI-native network slicing for 5G networks. Successful 5G deployments rely on the use and integration of many other new technologies. In this blog post, Tameem Sheble RAN data scientist at Digis Squared, and AI for 5G researcher, takes us on a deep dive through the close integration of 5G with AI, and how network slicing is vital to deliver the 5G vision.
The view from 2015: the 5G vision
New telecom technologies take years to be discussed, agreed and defined in standards. Previous wireless technologies have been designed and architected for one major use case: enabling mobile broadband.
During the development phase of 5G standards, by means of reaching consensus and aligning expectations, the International Telecoms Union (ITU) defined their framework and overall objectives of the future out to 2020 and beyond, in a vision document. As part of this workaround 5G architecture, they considered three distinctive, cutting-edge service verticals – enhanced mobile broadband, massive machine-type communications and ultra-reliable and low-latency communications – and their usage scenarios and opportunities for communications service providers (CSPs).
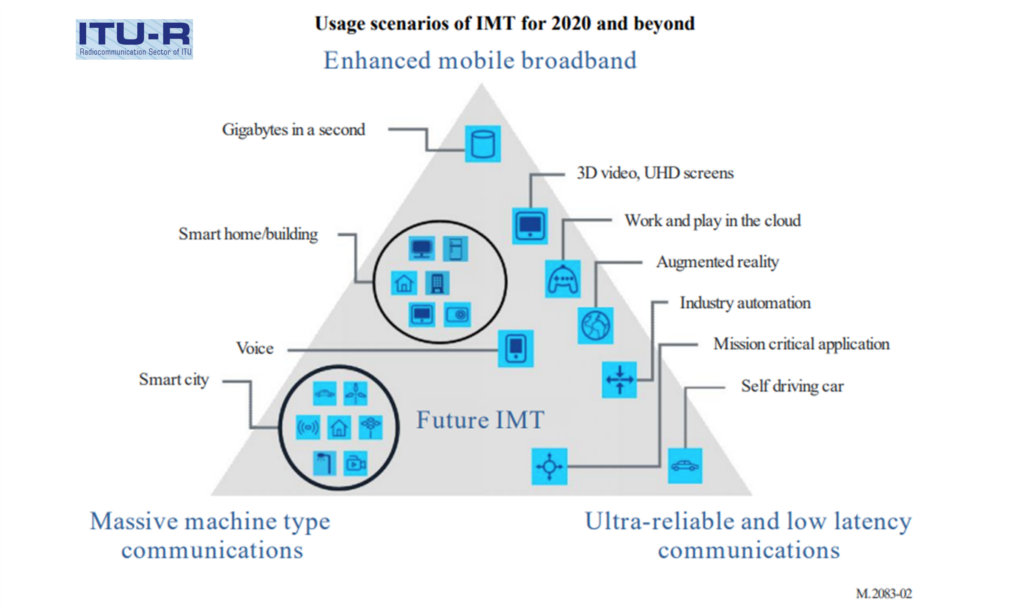
Network Slicing (NS) is one of the 5G key enablers. It’s a technique that CSPs can use to satisfy the different needs and demands of the 5G heterogeneous verticals, as illustrated below, using the same physical network infrastructure.
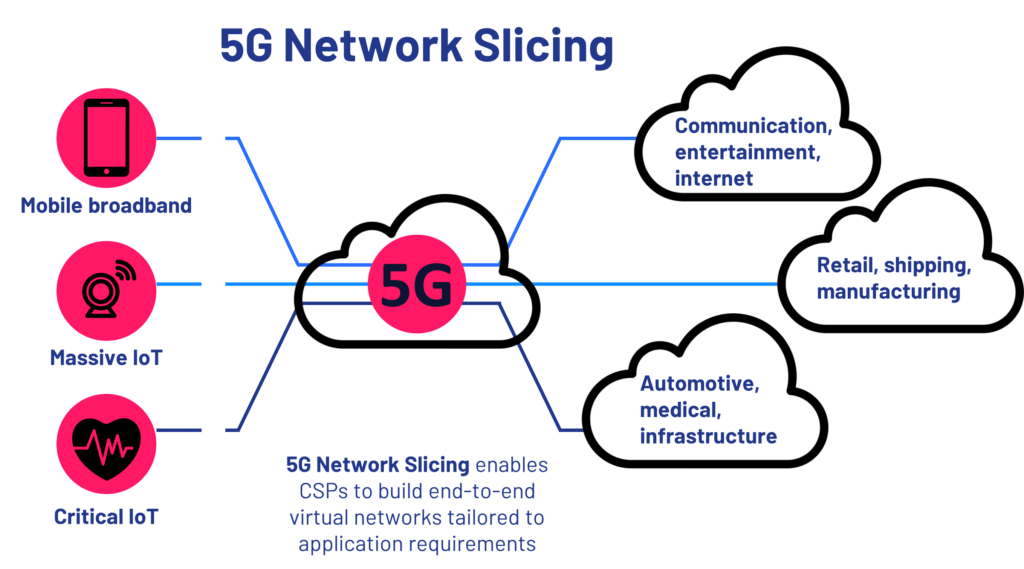
AI-native network slicing for 5G networks
Network slicing enables the virtual and independent logical separation of physical networks. It’s a technique used to unlock the value of 5G networks, by opening the possibilities for customer-centric services based on the demand on the network while managing cost and complexity. Consequently, vendors and standardization communities consider 5G NS a key paradigm for 5G and beyond mobile network generations.
Although the 5G NS process brings flexibility, it also increases the complexity of network management. The introduction of AI into the 5G architecture, AI-native, is motivated by the vast amount of unexploited data and the inherent complexity and diversity that requires AI to be deployed as an integral part of the overall system design. Although the rising temptation is to rely on AI as a pillar for managing 5G network complexity, in practical terms, AI and 5G are indivisible. They tend to converge from an application perspective, and they become two halves of a whole. AI’s value relies on 5G; for example; critical data-driven decisions need to be communicated with ultra-low latency and high reliability.
AI as a potential solution to network slicing
Let’s turn now to addressing AI in a nutshell for the management of the complex sliced 5G network, a complexity that relates to decision-making towards efficient, dynamic management of resources in real-time. CSPs need to leverage the use of the vast volume of data flowing through the network in a proactive way, by forecasting and exploiting the future system behaviour.
The management lifecycle of a network slice consists of four main phases,
- Preparation
- Instantiation
- Operation
- Decommissioning.
Many researchers have proposed AI solutions that underline the first 3 phases, as the decommissioning phase doesn’t involve management decisions. Admission control and network resources orchestration are some of the key slice management functions that need to make slice-level decisions to meet their requirements, while simultaneously maximizing the overall system performance.
Looking at this in more detail, this is achieved by controlling a massive number of parameters as a result of uncovering complex multivariate relationships that are related to each other in time, geolocation, etc. Proposing an AI solution must be done case by case depending on problem formulation and framing, algorithmic requirements, the scarcity and type of data and the operational time dynamics.
AI for network slices admission control (Phase 1)
Admission control – during the slice preparation phase – is a very critical decision-making control mechanism, it ensures that the requirements of the admitted slices are satisfied. During this control mechanism, a trade-off between resources sharing and KPIs fulfilment needs to be tackled. The decision on how many network slices run simultaneously, and how to share the network infrastructure between those slices, has an impact on the revenues of the CSPs.
The trade-off is further complicated by variables that alter over time, which makes the optimization of revenue based on admitted slices a difficult task. This is where Deep Reinforcement Learning (DRL) approach comes into the picture.
In a nutshell, the DRL algorithm has to learn the arrival pattern of network slices and make, for example, revenue-maximizing decisions based on the current system utilization and the anticipated long-term revenue evolution. Once a network slice is requested, and based on the system current utilization, two separate neural networks are in charge of scoring the two actions (i.e., accepting or rejecting the request), where each score represents the revenue associated with each action. Based on the difference in scores, the action corresponding to the higher revenue is selected; the algorithm interacts with the system and evaluates the accuracy of the forecasted revenue through a loss function. This value is then feedback to the corresponding neural network to perform weight update, so that the algorithm starts converging to a global maximum and performs better in the subsequent request iterations.
AI for network resources orchestration and re-orchestration (Phase 2 and 3)
After the successful admission, slices must be allocated sufficient resources in such a way that the available capacity is used in the most efficient way that minimizes the operational expenses (OPEX). The trade-off here is between under-provisioning that leads to Service Level Agreement
(SLA) violation, and over-dimensioning thus wasting resources.
CSPs need to be proactive by forecasting, at a slice level, the future capacity needed, based on previous traffic demand, and consequently timely reallocate resources when and where needed. This is where the Convolutional Neural Network (CNN) architecture comes into the picture for time-series forecasting.
Legacy state-of-the-art traffic time-series forecasting models focus on forecasting the future demand that minimizes some symmetric loss (e.g., mean absolute error), that treats both under- and over-prediction equally. But this type of legacy approach doesn’t consider the risk of under-provisioning and SLA violation – it is useless for 5G deployment!
Researchers argue that a practical AI-native resource orchestration solution has to forecast the minimum provisioned capacity that prevents SLA violation. The balance between over-dimensioning and under-provisioning is therefore controlled by the CSPs, by introducing a customized loss function that overcomes the drawbacks of the “vanilla symmetric losses”.
The AI literature proposes the use of 3-dimensional CNN architecture over the recurrent neural network (RNN) architecture – which is considered the state-of-the-art algorithm for forecasting time-series data – in order to exploit and uncover spatial and temporal traffic relationships.
The future for AI in 5G and beyond
Whilst general AI limitations are now well known – trustworthiness, generalization and interpretability – exploiting AI to assess and manage complex decisions is vital for the smooth operation of 5G networks. And as network technologies continue to grow in complexity and capability, AI will clearly be necessary as a pillar technology for future-generation zero-touch mobile networks
In conversation with Tameem Sheble RAN data scientist at Digis Squared, and AI for 5G researcher.
If you or your team would like to discover more about our capabilities, please get in touch: use this link or email sales@DigisSquared.com .
Discover more
Digis Squared, independent telecoms expertise.
References
- IMT Vision – Framework and overall objectives of the future development of IMT for 2020 and beyond (2015) – Figure 2, page 12, Usage scenarios of IMT for 2020 and beyond