Leveraging advanced analytics and AI engine, IPM predicts and prevents network performance issues before they happen, building Capacity growth models and forecasting user behaviour and traffic load on the network, giving proper recommendations that keep network performance on track with this growth and user behaviour changes.
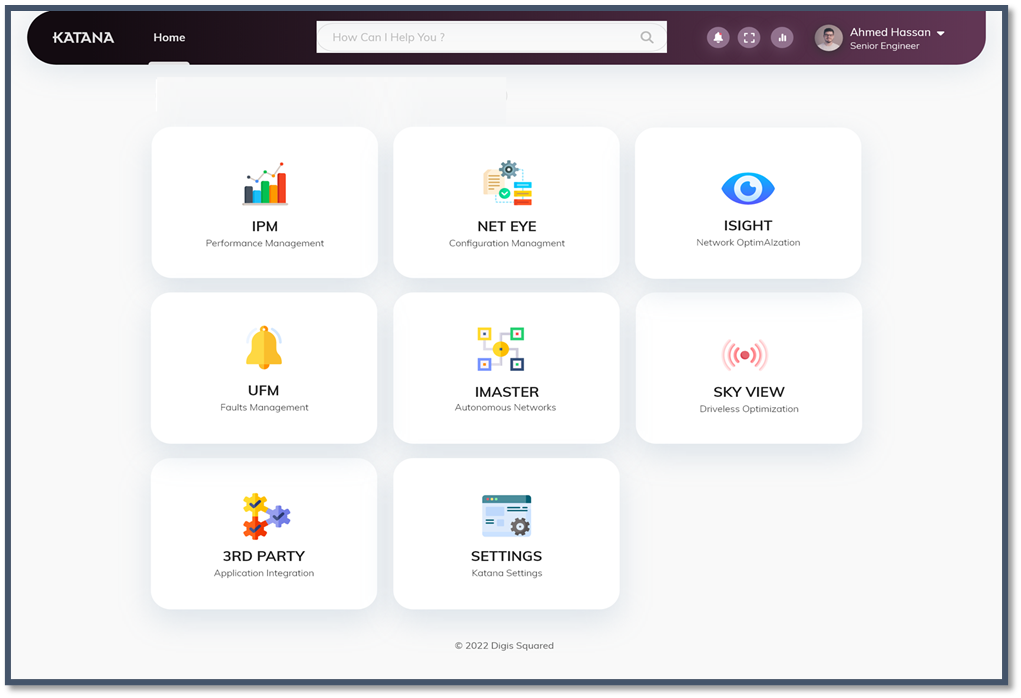
IPM Analytics is the heart of KATANA IPM module, and it offers the below different uses cases.
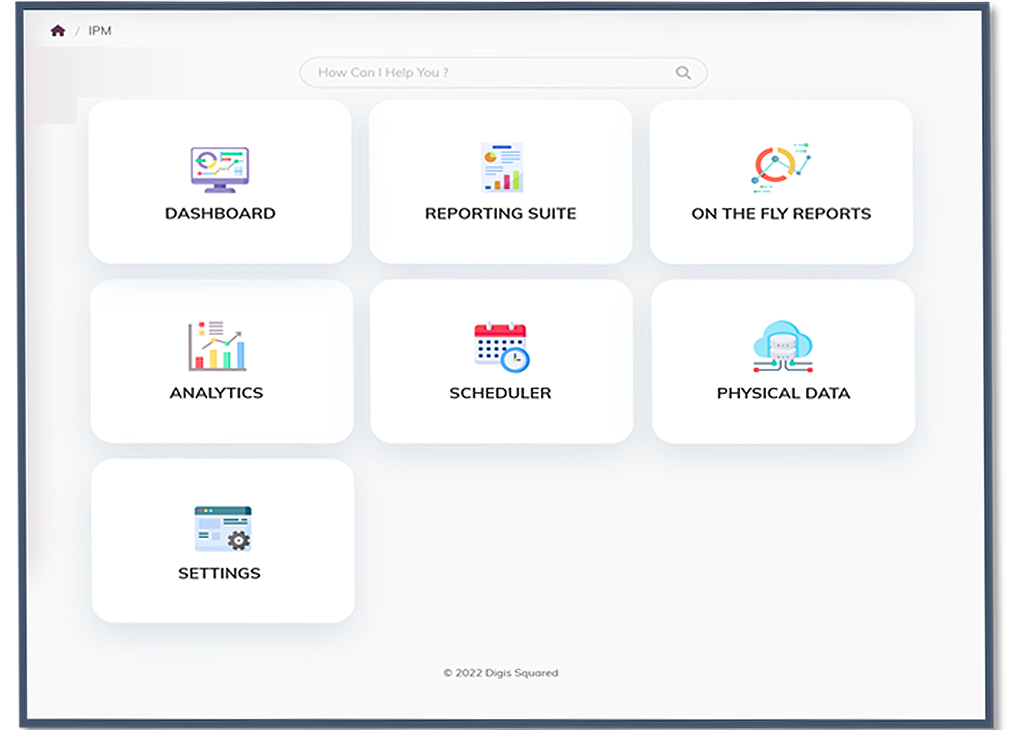
Anomaly Detection:employs a machine-learning algorithm to understand the patterns of Key Performance Indicators (KPIs), making comparisons and autonomously recognizing deviations. The resulting scores are presented for each instance, facilitating straightforward identification of anomalies and their deviation from the typical cluster or common behavioral patterns within the network.
Forecasting Analysis: iPM encompasses various forecasting techniques within a unified interface, granting users the capability to analyze anticipated future trends in network usage for any counter and Key Performance Indicator (KPI).
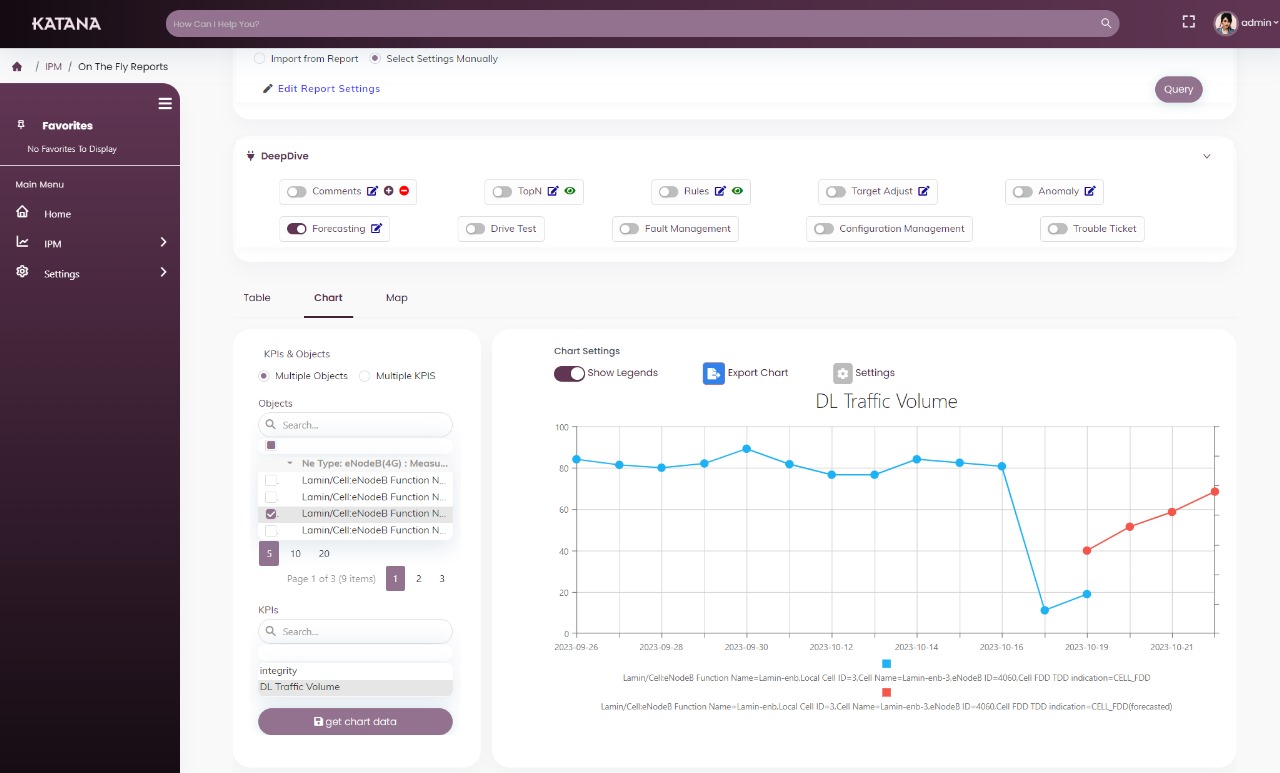
Capacity Management: As networks expand and experience heightened traffic, there is often a decline in network performance. To prevent this deterioration, iPM Capacity management function becomes crucial to enhance performance and restore it to its initial levels. iPM is Addressing traffic shifts requires the strategic rebalancing of network traffic, ensuring even utilization across the network, thereby deferring capital expenditures on new equipment.
Worst Cell List: The Worst Cell List Report, an integral component of our iPM capabilities, is robustly supported by ranking conditions tied to specific periods for designated Key Performance Indicators (KPIs). This functionality empowers users to assess nodes with the poorest performance through detailed maps and charts.
Worst Degraded List: This module, seamlessly integrated into our iPM suite, efficiently troubleshoots and compiles a list of nodes with degraded performance over a specified period. It conducts in-depth analyses through maps and charts, facilitating immediate examination at the work area for detailed troubleshooting
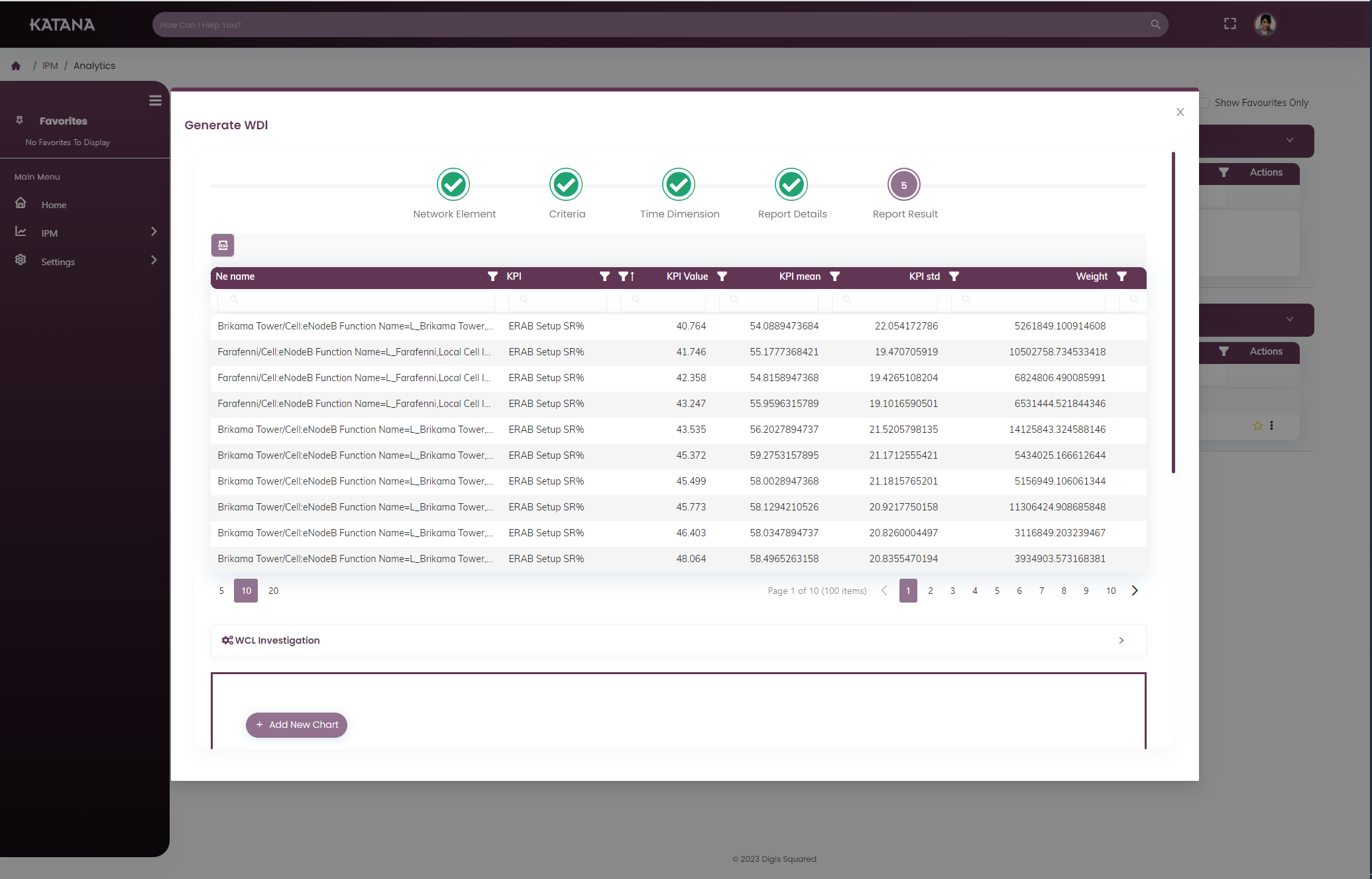
Benchmark: After implementing an optimization action, users have the flexibility to initiate a benchmark across a set of Key Performance Indicators (KPIs). This benchmarking can be conducted on a Day-to-Day, Week-to-Week, or Month-to-Month basis, allowing for comprehensive performance evaluation over various timeframes.
Swap & Acceptance: In Swap Projects, users are required to compare Key Performance Indicators (KPIs) before and after the swap. iPM provides users with the convenient option to effortlessly compare the performance of vendors, facilitating a streamlined assessment of the impact of the swap on network performance.